Spatial transcriptomics (ST) is a groundbreaking method that allows scientists to measure gene activity in a tissue sample and retain spatial information. However, most spatial ST technologies are limited by their resolution. To improve the resolution, many existing methods combine single-cell RNA sequencing (scRNAseq) data and ST data and try to deconvolute the ST data. Although the cell type proportion in one spot can be inferred from the existing method, the gene expressions of every single cell in spots are still unknown. In the seminar, we propose a method that can generate single-cell gene expressions in spots using scRNAseq data as the reference data and thus realize the actual single-cell resolution spatially-resolved transcriptomics. In the model, we use score-based generative models to learn the distribution of reference scRNA-seq data. We demonstrate that the model outperforms existing methods for estimating cell-type proportions and single-cell gene expressions using simulations. Applied to mouse brain ST data, our model can provide more reliable high-resolution gene expressions.
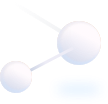
HKUST
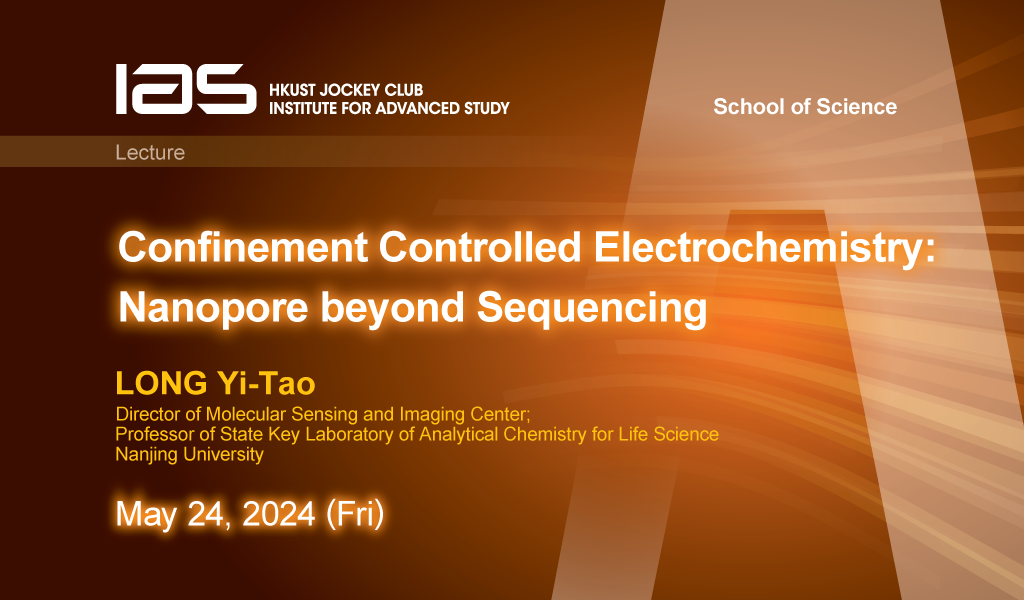
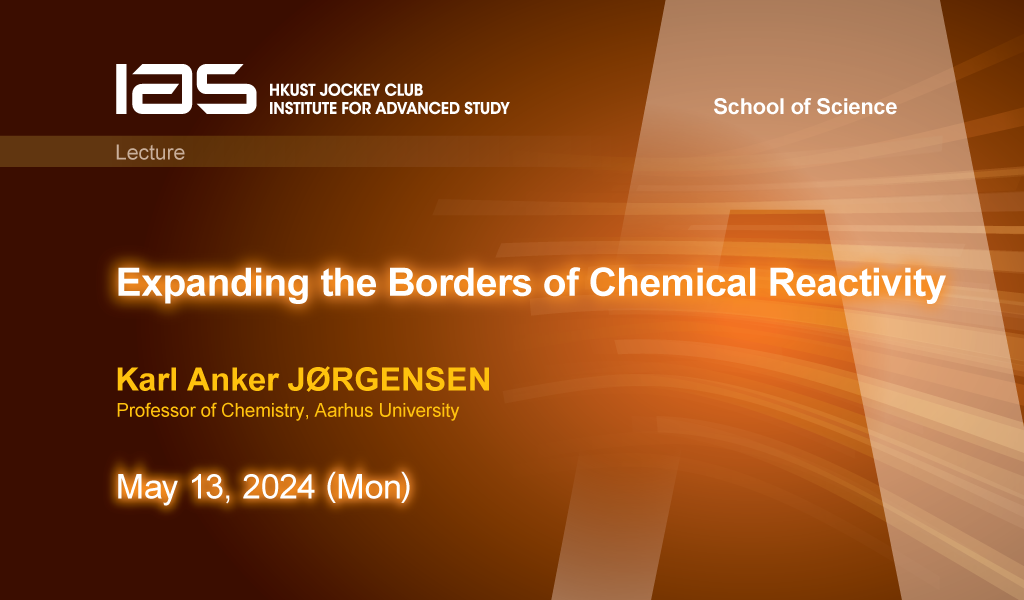