Policy gradient (PG) methods and their variants lie at the heart of modern reinforcement learning. Due to the intrinsic non-concavity of value maximization, however, the theoretical underpinnings of PG-type methods have been limited even until recently. In this talk, we discuss both the ineffectiveness and effectiveness of nonconvex policy optimization. On the one hand, we demonstrate that the popular softmax policy gradient method can take exponential time to converge. On the other hand, we show that employing natural policy gradients and enforcing entropy regularization allows for fast global convergence.
17 Oct 2022
11am - 12pm
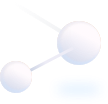
Where
https://hkust.zoom.us/j/94883840530 (Passcode: hkust)
Speakers/Performers
Prof. Yuting WEI
The Wharton School, University of Pennsyvania
The Wharton School, University of Pennsyvania
Organizer(S)
Department of Mathematics
Contact/Enquiries
Payment Details
Audience
Alumni, Faculty and staff, PG students, UG students
Language(s)
English
Other Events
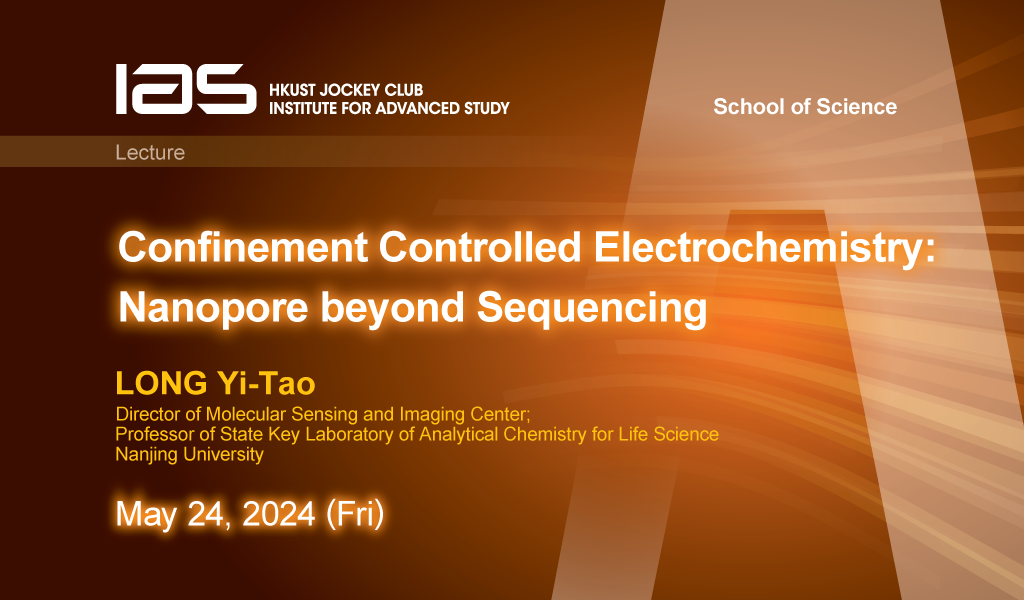
24 May 2024
Seminar, Lecture, Talk
IAS / School of Science Joint Lecture - Confinement Controlled Electrochemistry: Nanopore beyond Sequencing
Abstract
Nanopore electrochemistry refers to the promising measurement science based on elaborate pore structures, which offers a well-defined geometric confined space to adopt and characterize sin...
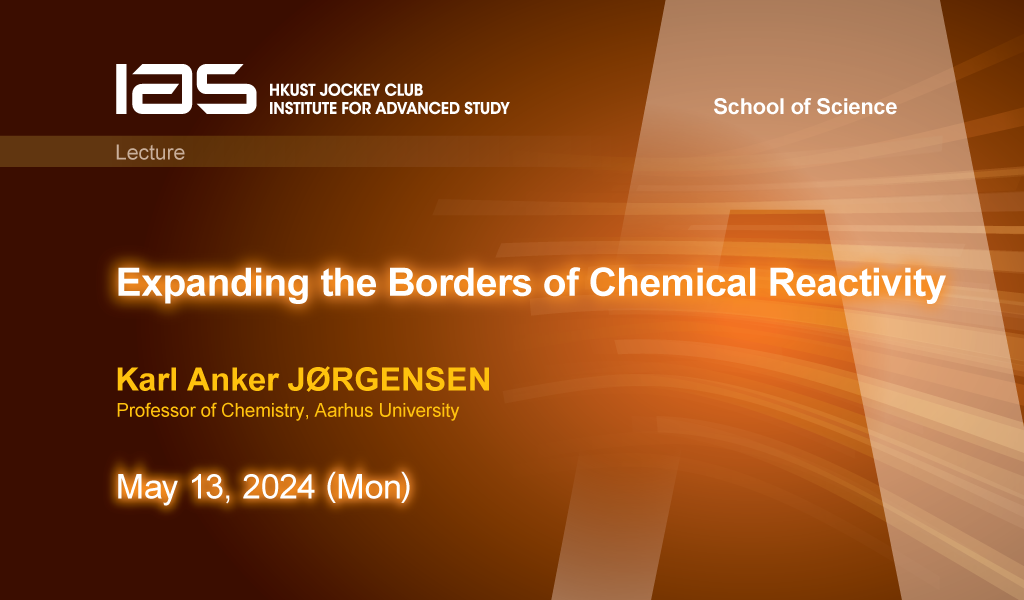
13 May 2024
Seminar, Lecture, Talk
IAS / School of Science Joint Lecture – Expanding the Borders of Chemical Reactivity
Abstract
The lecture will demonstrate how it has been possible to expand the borders of cycloadditions beyond the “classical types of cycloadditions” applying organocatalytic activation principles....